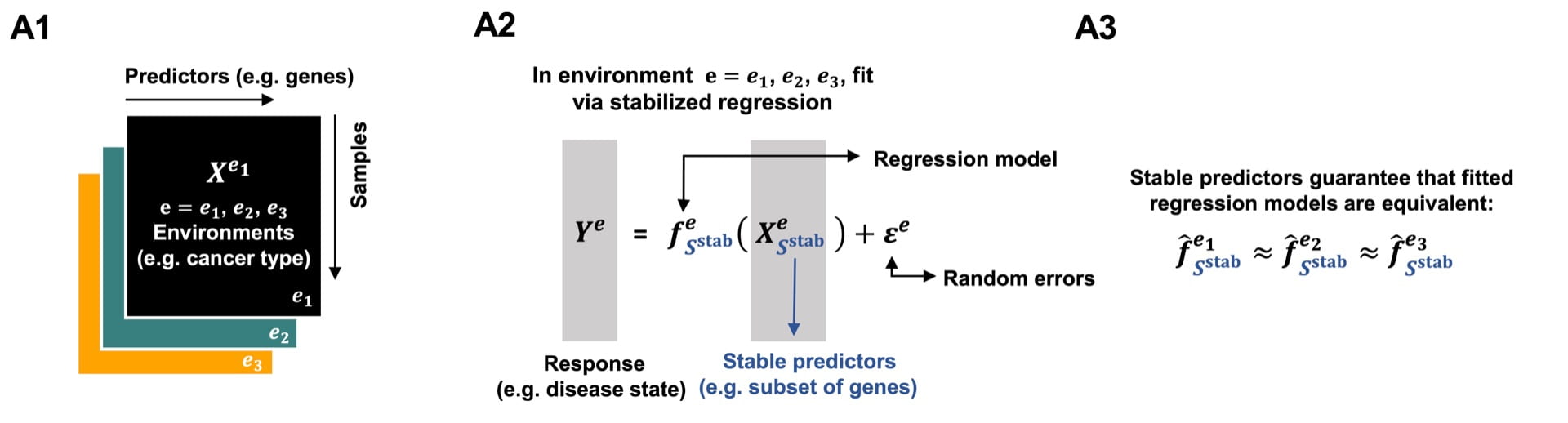
StableMate to select stable predictors in omics data
Variable selection in ‘omics data is essential to identify reliable markers of diseases, or shed light into molecular mechanisms. however, many variable selection methods in multivariate analysis (e.g. lasso or elastic net penalised regression, machine learning approaches) lack of robustness against small variation in the data.
PhD student Yidi Deng was inspired by the stabilised regression framework from Pfister et al. (2021) that enables to distinguish stable and unstable variables across different experiments or environments. Stable features are reproducible and generalisable to new experiments or environments (forging new avenues towards the discovery of causal relationships), while unstable features inform about biological characteristics of particular experiment. The overarching aim is to build a model using stable predictors that are generalisable to unseen environments.
In StableMate, we have addressed critical computational issues in the SR framework with a new version of stochastic stepwise regression (adapted from Xin et al. 2012). We have improved the accuracy of the generalisable model. StableMate is a flexible framework which we have applied for different types of analysis (regression and classification), and different types of data (bulk RNA-seq, metagenomics and single cell RNA-seq).
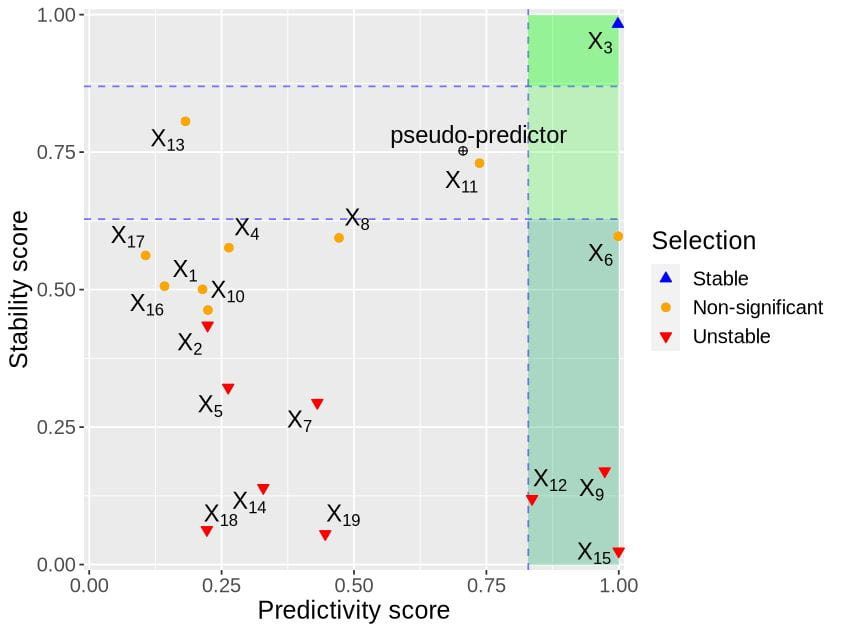
, , ,
Pfister, N., Williams, E. G., Peters, J., Aebersold, R. & Bühlmann, P. (2021). Stabilizing variable selection and regression. The Annals of Applied Statistics, 15(3), 1220-1246.
Xin, L., & Zhu, M. (2012). Stochastic stepwise ensembles for variable selection. Journal of Computational and Graphical Statistics, 21(2), 275-294.
Categories