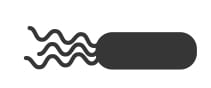
Managing batch effects in microbiome studies
Yiwen (Eva) Wang’s first publication from her Ph.D studies was published in Briefings in Bioinformatics. In her article review, Eva describes the topical issues of batch effect in microbiome studies. Sources of batch can be technical, but also computational and biological. Most methods assume batch effect are systematic across all batches. Interestingly, by analysing several data sets, we found out that it was not the case, as specific microbial variables seem to be more affected than others to the effect of batch.

We reviewed methods that either correct, or account for batch effects, but most were specifically developed for RNA-sequencing data, and thus are limited for microbiome studies that have inherent data characteristics (sparse, compositional, multivariate). We provide practical guidelines for assessing the efficiency of the methods based on visual and numerical outputs and a thorough tutorial to reproduce the analyses conducted in this article.
Key points from the article:
- Batch effects originate from biological, technical and computational sources.
- Microbiome data have inherent data characteris- tics, including sparsity and over-dispersion, uneven library sizes, compositional structure and inter-variable dependency.
- Caution is needed when selecting batch effect adjust- ment methods because of their strong assumptions: current methods are borrowed from genomic expres- sion research, whose data characteristics differ from microbiome data.
- Preserving the effects of interest while correcting for batch effects is of primary importance and should be assessed via visual and numerical tools, before and after batch effect correction.
Yiwen Wang and Kim-Anh Lê Cao (2019) Managing batch effects in microbiome data, Briefings in Bioinformatics in press doi: 10.1093/bib/bbz105
Categories